Using Machine Learning to Predict Student Outcomes
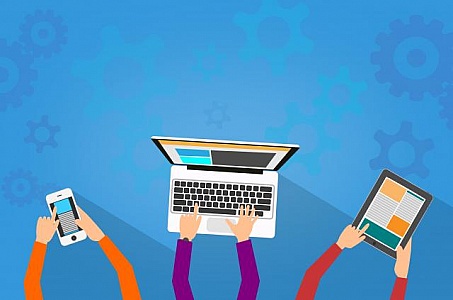
Student retention remains one of the greatest concerns for higher education institutions worldwide. In the U.S. only about 60% of students graduate within six years. Retention rates are used as an indication of how many undergraduate students who start their studies at a college will continue their studies that at that college.
Many factors play a role in early withdrawal from university, mostly to do with financial and personal reasons. Universities and colleges also lose students when they decide to study at other schools, or take online courses at other schools or decide to take their sophomore year off.
Technology in the form of machine learning is being used by universities to improve their student support and retention rates. The value of machine learning for the improvement of retention rates lies in its predictive power. Machine learning algorithms are able to analyze vast data sets and identify students who are at risk of abandoning their studies.
For instance, it’s a well-known fact that students who do poorly academically are less likely to graduate. It’s also possible that poor grades in a specific class can be a precursor to a student dropping out of that particular class as well as the entire course.
This is exactly the type of trend that predictive analytics used by Georgia State was able to uncover. The university learned that nursing students who did poorly in Introductory Math did not succeed in completing their course – only 10% of the students who got a C or lower in their math went on to graduate. Of the students who achieved B+ or better, 80% graduated.
Georgia State sprang into action, increasing its number of student advisors. This simple step led to an increase in both its four-year graduation rate and six-year graduation rate by 5% and 6% respectively.
The University of Arizona used machine learning to leverage data that go beyond course grades and demographics. UA is taking the matter a step further by accessing location and transactional data from student swipe cards. This gives the university a perspective on the wider context of students’ lives. The thinking is that machine learning will help identify which factors relate to student retention.
There’s another reason for broadening the range of information gathered on students beyond information on their grades. The fact is that freshmen who abandon their studies do so for reasons that may have nothing to do with grades, as they usually decide to leave the university in the first twelve weeks before they have received their grades.
The sooner the university finds out what these reasons are, the sooner it can implement intervention measures.
Southern Connecticut State University teamed up with IBM on the issue of student retention. The university has integrated IBM Watson Analytics to improve students’ academic achievement and development. IBM’s Watson Analytics Professional is able to gain insights from many student data points to help the university retain a higher percentage of students.
Watson Analytics helped SCSU to make the unexpected discovery that students’ experiences on campus accurately predicted whether they would graduate from the university, transfer to another institution or terminate their education.
The university used this information to design various programs, the most successful of which is the Academic Success Center, which provides services that support students to become independent scholars and achieve academic success.
Insights from machine learning are helping tertiary institutions to attain higher retention rates and in the process also help students to be more successful in their studies.