Diffusion Is Spectral Autoregression
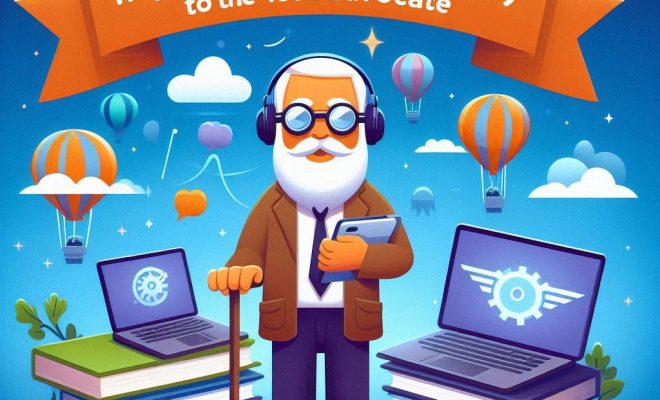
The world of generative models has been revolutionized by the rise of diffusion models, capable of creating stunningly realistic images, videos, and even audio. While these models have achieved impressive results, their internal workings remain a bit of a mystery. Recent research, however, has shed light on the relationship between diffusion and a more familiar concept: spectral autoregression.
Spectral autoregression is a powerful tool for analyzing and modeling time series data. It involves representing the signal as a combination of sinusoidal waves, each with a specific frequency and amplitude. By understanding the spectral properties of the data, we can build models that predict future values.
The key insight lies in recognizing that the diffusion process itself can be interpreted as a form of spectral autoregression. As a diffusion model gradually adds noise to the data, it essentially introduces a spectrum of frequencies into the signal. The reverse process, where the model learns to remove the noise, can be seen as a spectral autoregression process, reconstructing the original signal from its spectral representation.
This new understanding has several exciting implications. Firstly, it provides a deeper theoretical foundation for diffusion models, helping us understand their behavior and limitations. Secondly, it opens up new avenues for developing more efficient and powerful diffusion models. By incorporating spectral techniques, we can potentially design models that learn faster and generate higher-quality outputs.
Finally, the link between diffusion and spectral autoregression can lead to novel applications in various fields. For instance, we can leverage this knowledge to analyze and model complex time series data, improving forecasting and anomaly detection in areas such as finance, healthcare, and climate science.
In conclusion, the revelation that diffusion is spectral autoregression represents a significant breakthrough in our understanding of generative models. By bridging the gap between these seemingly disparate concepts, we unlock new possibilities for research, development, and practical applications. This exciting discovery is sure to pave the way for even more powerful and innovative generative models in the future.