Automation: The Future of Data Science and Machine Learning?
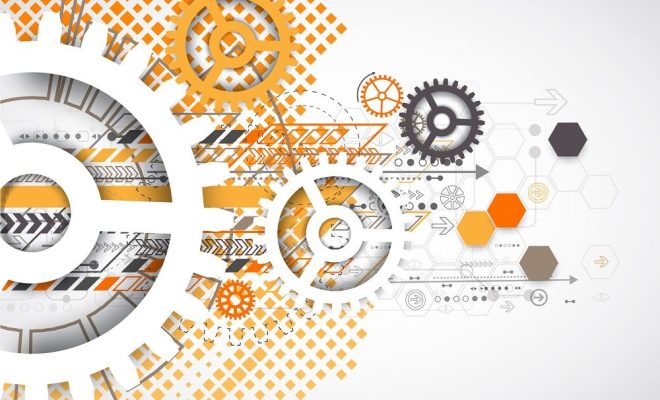
Automation has been revolutionizing numerous industries in recent years, and Data Science and Machine Learning haven’t been left out of the race. Automation has enabled data science teams to speed up and streamline their workflow, resulting in saving time and resources. Not only that, but automation has also optimized machine learning processes, leading to better data-driven decisions. In this article, we’ll explore how automation can further propel data science and machine learning and why it’s the future of these fields.
Automating Data Cleaning & Preparation:
Data cleaning and preparation constitute a significant chunk of any data science project. With automation, this step can be speed up by identifying and cleaning up missing values, anomalies and standardization of data. Data can be pulled from various sources and loaded into the system. Moreover, automation can also help shrink the time-consuming process of data labeling, extract relevant information, and even transform unstructured data.
Reducing Human Bias:
One of the significant advantages of automation is reducing human bias. Machine learning algorithms rely on data to make decisions. If the data is biased, the algorithms will also be biased. Automation can help reduce bias by ensuring that machine learning models are trained on unbiased data. It can also help identify where bias exists in data and model, enabling the users to correct for it.
Automating Feature Engineering:
Feature Engineering is the practice of selecting and transforming raw data into meaningful predictors. With automation, feature engineering can be speed up by employing Machine learning heuristics and AI-assisted feature selection to identify which features are more relevant to the model. Besides, automating feature engineering can help reduce redundant features and collinearity, which can negatively impact the model’s predictive power.
Predictive Modeling:
Automation can exponentially increase model selection and tuning efficiency. For example, techniques such as Bayesian optimization can help to automate the model selection process, identifying the best performing models, and hyperparameters. Automation can also be used to automatically handle missing or invalid data and automatically retrain the model with new data to improve accuracy.
Scalability:
As datasets and business complexity increase, data science and machine learning teams will have to scale their efforts. Automation can help streamline the process, reduce costs, and increase efficiency. By automating tasks like data preparation, data cleaning, feature engineering, and model selection, data science teams can focus on the more complex tasks while lowering the risk of errors.
Conclusion:
Automation is rapidly becoming the future of data science and machine learning in the digital age. Automation will optimize workflows, enable reduction of human bias, and above all, scale efforts as data complexity and volume grow. The key takeaway is that organizations must embrace automation to achieve competitive advantage and improve their analytical capabilities. Furthermore, Automation must be viewed not as a replacement for human capital but an augmentation of it to generate more value for business.